Explore the Future trends in predictive analysis include the integration of AI and machine learning, leveraging big data for real-time insights, and its expanding role in healthcare for better patient outcomes and operational efficiency.
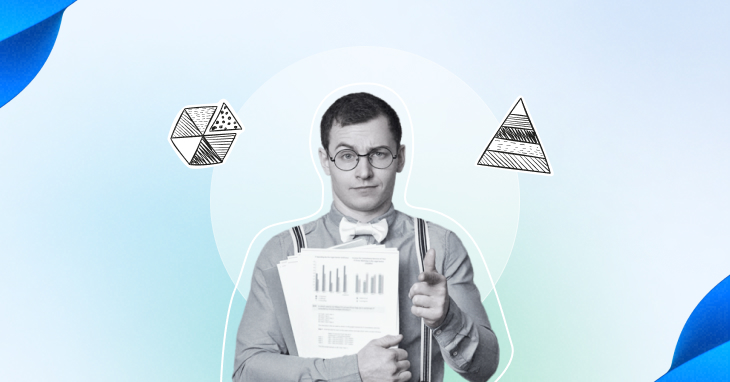
Predictive analysis is one of the most powerful tools at businesses’ disposal in today’s data-driven world, as they are constantly seeking ways to gain a competitive edge. It involves using data, statistical algorithms, and machine learning techniques to identify the likelihood of future outcomes based on historical data.
As technology continues to advance, the future of predictive analysis promises exciting developments that can revolutionize industries across the board.
What is Predictive Analysis?
Predictive analysis is a data analytics technique that uses historical and current data to forecast future outcomes with a high degree of accuracy. It involves the use of statistical algorithms, machine learning models, and data mining to identify patterns, trends, and relationships within datasets.
By analyzing past data points and their relationships, It help businesses make informed decisions, anticipate trends, mitigate risks, and capitalize on opportunities.
This powerful tool finds applications across various industries, including finance, healthcare, marketing, and more. In finance, predictive analysis can be used to predict stock prices, detect fraudulent activities, and manage risks.
In healthcare, it aids in forecasting patient outcomes and identifying potential disease outbreaks. Marketing departments rely on predictive analysis for targeted marketing campaigns, customer segmentation, and predicting customer behavior.
Overall, predictive analysis enables organizations to gain valuable insights into their operations, customers, and markets. By leveraging these insights, businesses can optimize processes, enhance customer experiences, and ultimately drive growth and competitiveness in today’s dynamic business environment.
Understanding Predictive Analysis
Before delving into the future trends of predictive analysis, it is essential to understand its core principles and applications. Predictive analysis utilizes a variety of statistical techniques, machine learning algorithms, and data mining to analyze current and historical facts to make predictions about future events.
This valuable tool helps businesses make informed decisions, mitigate risks, identify opportunities, and optimize operations.
Key Components of Predictive Analysis
enhance customer experienceand Preparation: The first step in predictive analysis involves gathering relevant data from various sources. This data may include historical records, customer information, market trends, and more. Once collected, the data needs to be cleaned, transformed, and prepared for analysis.
Statistical analyses to enhance the customer experiences as regression analysis, time series analysis, and clustering are used to identify patterns, correlations, and relationships within the data.
Machine Learning Algorithms: Machine learning plays a crucial role in predictive analysis. Algorithms such as decision trees, random forests, neural networks, and support vector machines are utilized to build predictive models based on historical data.
Model Evaluation and Deployment: After developing the predictive models, they are evaluated using various metrics to ensure their accuracy and reliability. Once validated, these models are deployed to make predictions based on new data.
Applications of Predictive Analysis
Predictive analysis finds applications across diverse industries, including:
- Finance: Predicting stock prices, detecting fraudulent activities, and managing risk.
- Healthcare: Forecasting patient outcomes, identifying disease outbreaks, and personalized medicine.
- Retail: Analyzing customer behavior, optimizing pricing strategies, and inventory management.
- Marketing: Targeted marketing campaigns, customer segmentation, and predicting customer churn.
Now, let’s explore the future trends that are set to shape the landscape of predictive analysis in the coming years.
Future Trends in Predictive Analysis
AI-Powered Predictive Analytics
Artificial Intelligence (AI) is poised to revolutionize predictive analysis by enabling more sophisticated and accurate predictions. : Forecasting: Forecasting powered predictive analytics will leverage advanced algorithms and deep learning techniques to uncover hidden patterns in data.
This will lead to enhanced sales insights, better decision-making, and a deeper understanding of complex relationships within datasets.
Real-Time Predictions
The demand for real-time insights is driving the development of predictive models that can provide instant predictions. Businesses will increasingly rely on real-time predictive analytics to respond swiftly to changing market conditions, customer preferences, and emerging trends.
This trend will require advanced data processing capabilities and the integration of IoT (Internet of Things) devices to gather data in real-time.
Explainable AI
As predictive models become more complex, there is a growing need for transparency and interpretability. Explainable AI techniques will enable users to understand how a model arrives at a particular prediction. This transparency is crucial, especially in regulated industries such as healthcare and finance, where decisions can have significant consequences.
Automated Machine Learning (AutoML)
Automated Machine Learning (AutoML) will democratize predictive analysis by making it more accessible to businesses with limited data science expertise. AutoML platforms will automate the process of model selection, feature engineering, and hyperparameter tuning, allowing users to build accurate models with minimal manual intervention.
Predictive Analytics as a Service (PAaaS)
The rise of cloud computing has paved the way for Predictive Analytics as a Service (PAaaS) offerings. Businesses can now leverage cloud-based predictive analytics platforms to access powerful tools and resources without the need for extensive infrastructure. PAaaS solutions will enable organizations to scale their predictive analysis capabilities rapidly and cost-effectively.
Enhanced Data Security and Privacy
With the increasing volume of data being used for predictive analysis, there is a heightened focus on data security and privacy. Future trends will involve the implementation of robust data encryption techniques, secure data sharing protocols, and compliance with stringent data protection regulations such as GDPR (General Data Protection Regulation).
Augmented Analytics
Augmented analytics integrates AI and machine learning capabilities into business intelligence tools, enabling users to gain deeper insights from data effortlessly. This trend will empower business users with self-service analytics capabilities, allowing them to explore data, generate reports, and make data-driven decisions without relying on data scientists.
Integration of Predictive and Prescriptive Analytics
The convergence of predictive and prescriptive analytics will enable businesses not only to predict future outcomes but also to recommend the best course of action. By combining predictive models with optimization algorithms, organizations can make proactive decisions that drive desired outcomes and improve overall efficiency.
Conclusion
The future of predictive analysis is bright, with advancements in AI (Artificial Intelligence), real-time analytics, and automation poised to transform the way businesses operate. As organizations strive to gain a competitive edge, It will play an increasingly vital role in driving innovation, improving decision-making, and unlocking new opportunities.
Toolyt, a leading provider of predictive analytics solutions, stands at the forefront of this revolution.
By harnessing the power of cutting-edge technologies, Toolyt empowers businesses to harness the potential of their data, gain actionable insights, and stay ahead of the curve in an ever-evolving market landscape.
As businesses embrace the future of predictive analysis, they can rely on Toolyt to guide them on this transformative journey, enabling them to unlock the full potential of their data assets and drive sustainable growth in the digital age.